Artificial intelligence has come a long way since its inception. From Siri to self-driving cars, AI has infiltrated our lives in ways we never could have imagined. But how accurate is AI really? And who's to blame when it gets things wrong? Is it the algorithms, the training data, or the annotators who label the data? Let's take a look at the factors that affect the accuracy of AI products.
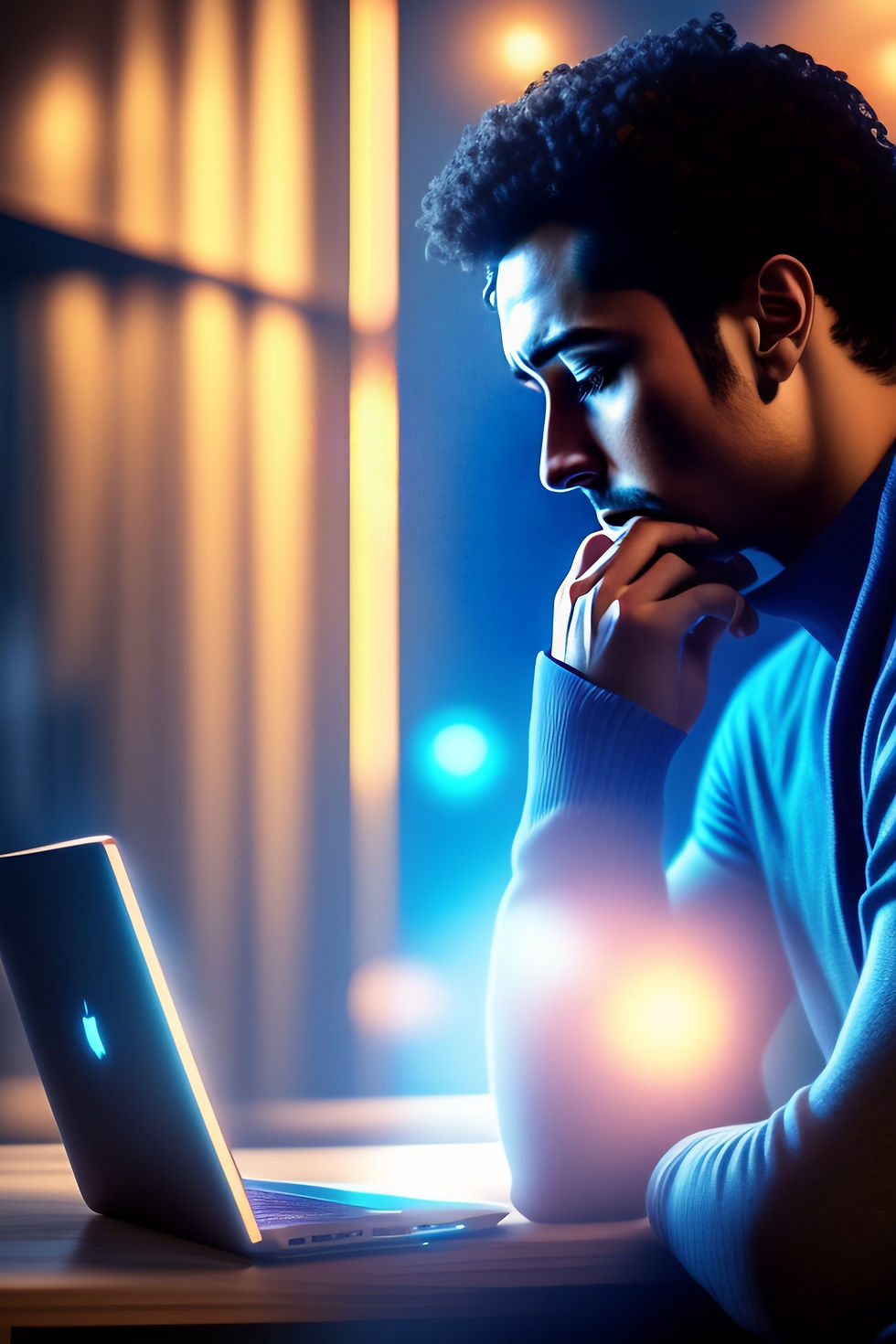
First up, we have the quality and quantity of training data. AI models need data to learn from, and the more data, the better, right? Well, not always. If the data is biased or incomplete, it can lead to inaccuracies in the AI product. So, we need high-quality data, but what does that mean? Is it data that's never been spilled on? Data that's never seen a day of humidity? Data that's never had its heart broken? The possibilities are endless. Just make sure the data is clean, unbiased, and plentiful, and you're on the right track. Next, we have model architecture and algorithm. These are fancy terms for the way the AI is designed and trained. Think of it like building a house. You need a solid foundation (architecture) and the right tools (algorithm) to build it. But what happens when you use the wrong tools? You end up with a wonky house that looks like something out of a Dr Seuss book. And nobody wants that. So, make sure you're using the right tools for the job, or you'll end up with a house of cards (pun intended). Now, let's talk about annotation quality and consistency. This is where the annotators come in. Annotators are the people who label the data used to train the AI model. But what if they get it wrong? What if they label a cat as a dog or a bird as a plane? Chaos, that's what. We need accurate and consistent annotations, or we'll end up with an AI product that's as confused as a chameleon in a bag of Skittles. So, to all the annotators out there, make sure you're labelling things correctly, or you'll be the reason why the robots take over (just kidding, probably). Moving on, we have changes in the data distribution. This is a fancy way of saying that the data used to train the AI model may not reflect real-world data. What if the AI is trained on data from a different time or place than where it's being used? It's like trying to teach someone how to drive a car from the 1950s. It may work, but it won't be pretty. We need to ensure the data used to train the AI model is up-to-date and relevant, or we'll end up with an AI product as useful as a screen door on a submarine. Lastly, we have external factors. These are the things that are out of our control, like changes in the environment, new regulations, or unforeseen events. It's like trying to plan a picnic in the middle of a hurricane. Sure, you could try, but it probably won't end well. We need to be prepared for the unexpected, or we'll end up with an AI product that's as reliable as a weatherman on April Fool's Day. In conclusion, there are several factors that affect the accuracy of an AI product, and they're all important. We need high-quality data, the right model architecture and algorithm, accurate and consistent annotations, relevant data distribution, and a plan for unexpected external factors. So, let's make sure we're building AI products that are accurate, reliable, and, most importantly, don't try to take over the world. After all, we don't want to end up like the humans in the Terminator movies, do we?
Comentarios